What Is “Experience-Based Robotics” That Realizes Intelligent Robots?
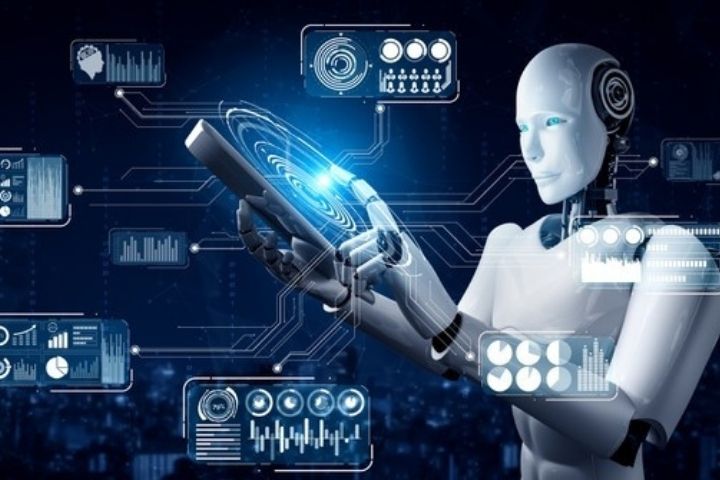
A professor at Waseda University Faculty of Science and Engineering and serves as a specific fellow at the Artificial Intelligence Research Center, National Institute of Advanced Industrial Science and Technology. Under the theme of “intelligence and industry of robots that deep learning innovates,” Ogata et al.
Have promoted a frame of predictive learning, which is a prediction based on data (experience) that integrates sensation and behavior and correction in real-time. He gave a lecture on the concept of “experience-based robotics” based on work and research cases with companies.
A System That Learns And Adapts In The Real World
The results obtained by deep learning have come to be seen in various forms. Many of them are capable of smoothly generating and recognizing images, videos, and sounds. The result leads to recognition of natural language and is being utilized in the dialogue between machines and humans.
However, most of the satisfactory performance is achieved by connecting a computer such as a PC or a cloud to a network. In fact, there are few cases where robots utilize deep learning in a stand-alone state. Still, it is quite difficult for robots to achieve the same intelligence as humans. “It’s not so easy to connect and transfer between the cyber and physical worlds. I’ve always been aware of where those gaps are,” Ogata said. He points out that “thinking about a system that learns and adapts in the real world, not just deep learning, may be an opportunity to think about the next AI (artificial intelligence).”
Currently, image recognition is becoming widely used as a function of robots that utilize deep learning. Image recognition technology is used for the degree of damage to objects and quality inspection. However, the difficulty in further evolving robot development is to incorporate the measurement results of sensors in the physical world into the model.
Therefore, a method of interpreting the sensor value and directly connecting it to the action without using a model was considered. A reward value is prepared for the difference between the robot’s sense (sensor value) and the action taken based on it.
Reinforcement learning to learn higher strategies that give higher rewards by giving higher rewards to well-resulting sensory and behavioral combinations is currently the focus of attention in the robot industry. There is. However, it is quite difficult to create a reward function that is the basis for judging “good” or “bad”, and the number of learnings is tens of thousands to hundreds of thousands, which is quite large.
Since it is difficult to perform this enormous number of learnings on an actual machine, simulations will be used, but evaluation of the analytical model created from this simulation is also an issue. For Go and Shogi, optimization can be done by setting the reward function, but for robots that work in the real world, it is difficult. Basically, when trying to predict, it fails, so it is necessary to first learn the result of the failed action and then change the internal state that generates the action.
Also Read : 6 ways To Take Screenshots Of Your Windows 10 Computer